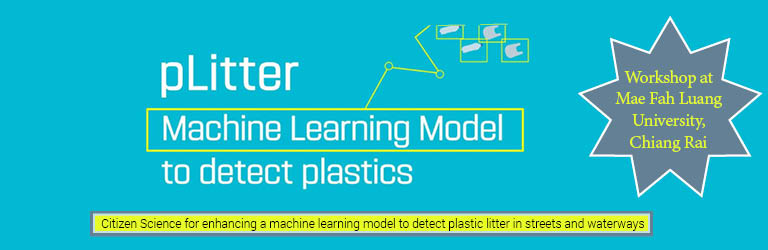
Chiang Rai, Thailand – (9/11) The Geoinformatics Center of the Asian Institute of Technology (GIC-AIT) held an interactive workshop at Mae Fah Luang University (MFU – Chiang Rai, Thailand) to introduce pLitter, a new online platform for enhancing a machine learning model to identify plastic litter in streets and waterways with citizen science.
GIC-AIT showcased pLitter’s plastic litter annotation capabilities for a group of 140 students from Dr. Panate Manomaivibool’s “Products and Environmental Impact” class in the Natural Resources and Environmental Management Program. The workshop was jointly organized by GIC-AIT and MFU under the United Nations Environment Programme (UNEP) CounterMEASURE Project, an initiative tackling regional marine plastic pollution. Dr. Manomaivibool opened the workshop by addressing new challenges to in-situ data collection brought on by the COVID-19 pandemic and the novel approach offered by GIC-AIT to address this issue. He encouraged his students to become agents of change with their participation in the workshop.
Tsukiji Makoto from UNEP briefly introduced CounterMEASURE and its objectives to reduce plastic pollution in the Asian region. He noted that rivers are the primary pathways for plastic litter and can negatively affect water bodies downstream. As the Mekong River is one of the region’s prominent natural resources, there is a call to action to prevent plastic litter from spoiling it. Therefore, a preventative measure was introduced through CounterMEASURE which integrates human contribution through citizen science with the novelty of technology in machine learning.
Citizen Science and How It Improves pLitter
At the core of the workshop, Dr. Kavinda Gunasekara–leader of the GIC team for the CounterMEASURE project–introduced students to pLitter and the concept of tackling marine plastic litter with mapping technology. In this approach, GIC implements both citizen science and machine learning to produce a plastic litter identification model in which citizen scientists are directly involved in training the model to identify plastic litter. The resulting output is a map depicting plastic waste distribution covering an entire study area.
“There are two types of citizen science we have understood during the development of our plastic litter identification model. Type 1 involves creating the model prediction by contributing images of plastic waste in the environment to our online platform, pLitter. Type 2 requires marking instances of plastic waste in those contributed images which serve as training data for the model, a process called annotation,” Dr. Gunasekara said in his presentation. During the workshop, Dr. Gunasekara asked students to contribute as the second type of citizen science to understand the image annotation process in pLitter.
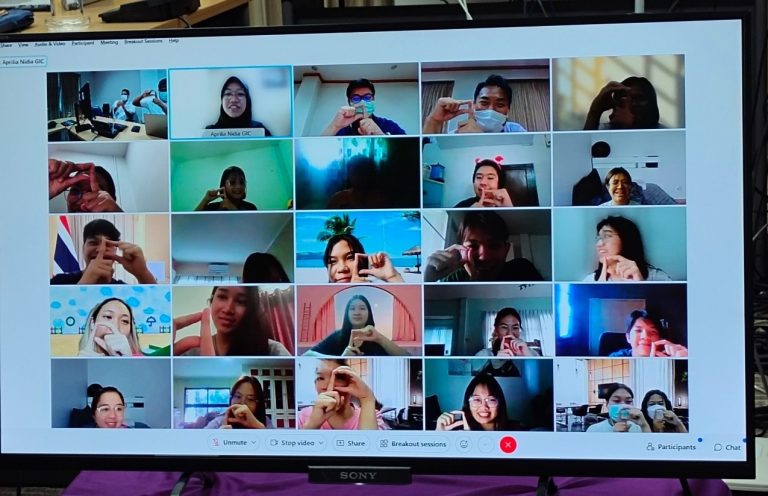
Participants Signing “P” for the pLitter Annotation Platform
High Enthusiasm for the Annotation Workshop
Although the participating students shared a background in environment, nearly half of the group previously had some exposure to machine learning in their university curriculum. Existing interest in the topic was met with enthusiasm to get involved in the annotation exercise.
There were 9 categories of objects to be annotated by the students: plastic bottles, clothes/textiles, facial masks, piles of litter, plastic bags, rope, single-use plastics (SUPs), trash bins, and wrappers/sachets. Led by GIC’s Sriram Reddy, the hands-on session began with a demonstration on the annotation process in pLitter followed by independent annotation by the students. In total, 2,830 images were reviewed during the workshop, with plastic annotated in 1,683 images (of the 2,830 images there were 1,147 without plastic in them) into the 9 categories, with plastics forming the majority of the annotations.
Prior to student plastic annotation, pLitter identified the following instances of plastic litter in the image dataset used in the workshop: plastic (10,345); piles (66); face masks (41); trash bins (19); and no instances of cloth/textiles, bottles, plastic bags, rope, single use plastics, nor wrappers/sachets. Following student image annotation, instances of all classes increased, especially prior zero instance classes. Student plastic image annotations were as follows: plastic (10,729); pile (119); face mask (66); trash bin (71); clothes/textiles (34); bottles (205); plastic bags (143); rope (35); single use plastics (60); wrappers/sachets (75). The increase in annotations from the workshop demonstrates the importance of training data for machine learning-based tools like pLitter. The new annotations will strengthen the plastic identification capabilities for incoming images from the region.
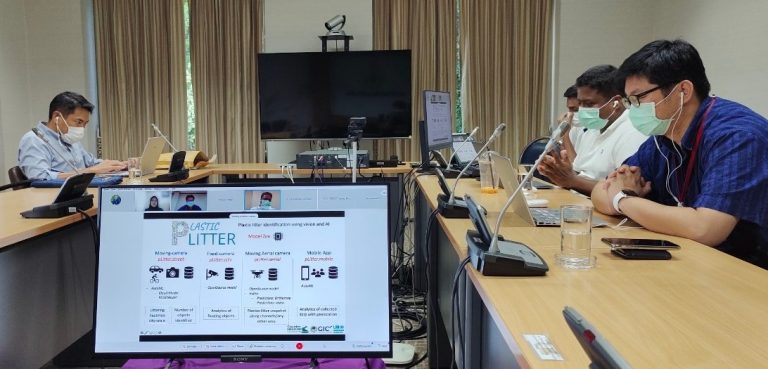
Annotation Workshop to detect Plastics led by Sriram Reddy
Launching the Contribution Platform for Plastic Pollution Branding Awareness
To spur model improvement and boost citizen scientist involvement, pLitter is also capable of receiving plastic litter image uploads where contributors can be involved in the Type 1 form of citizen science described by Dr. Gunasekara. Near the end of the workshop, Dr. Gunasekara presented the pLitter platform functionality to receive citizen scientist image uploads that were shot via smartphone. Citizen scientists then have the option to annotate their own images, thus contributing to enhancing the machine learning model for plastic litter identification.
With the objective for increasing the awareness of plastic pollution, Aprilia Rinasti – GIC’s moderator for the workshop – found that 43.2% of students who participated in the workshop were strongly aware of the issue and eager to contribute more. GIC-AIT is continuing to make improvements to pLitter through the end of 2021 for an enhanced user experience to gain wider audience support.
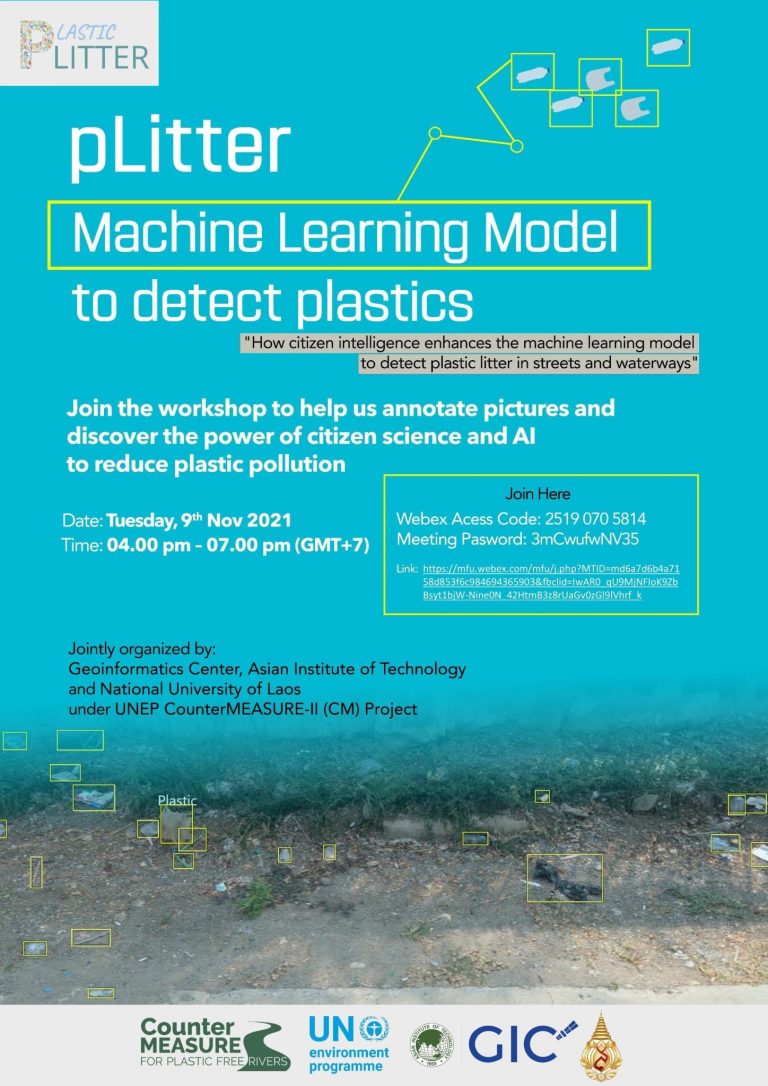
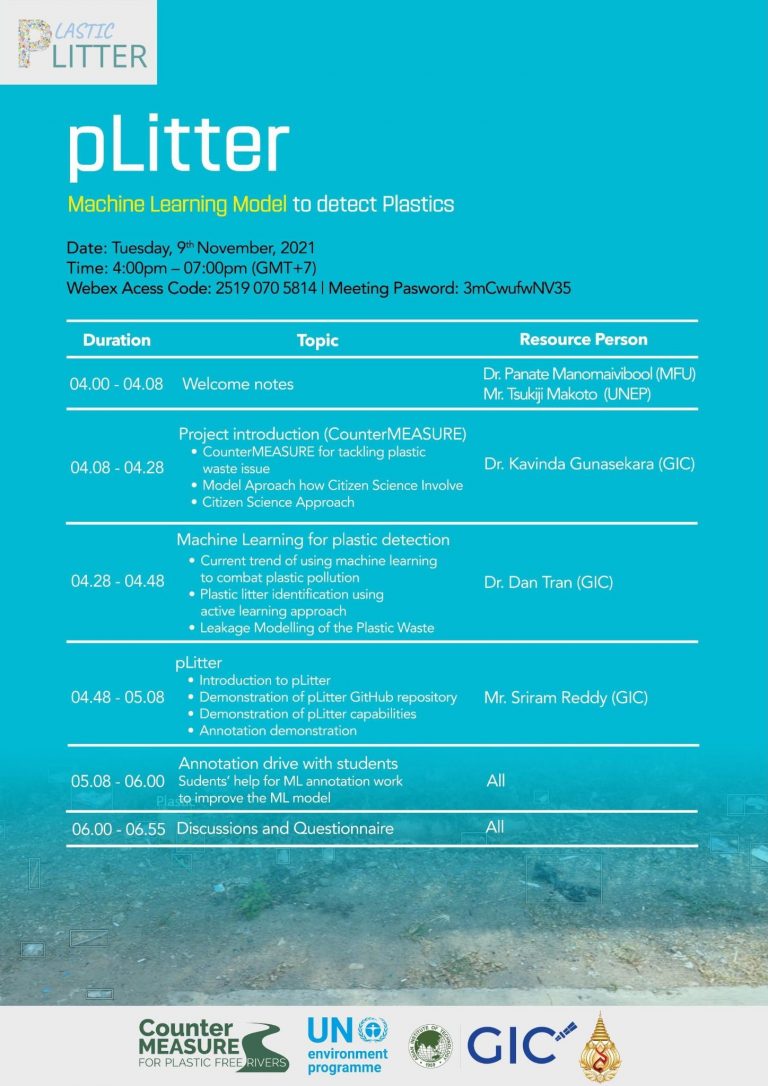